DiLiGenRT: A Photometric Stereo Dataset with Quantified
Roughness and Translucency
CVPR 2024 (Poster Presentation)
- Heng Guo 1,†
- Jieji Ren 2,†
- Feishi Wang 3,4,†
- Boxin Shi 3,4,‡
- Mingjun Ren 2,‡
- Yasuyuki Matsushita 5,‡
- 1School of Artificial Intelligence, Beijing University of Posts and Telecommunications
- 2School of Mechanical Engineering, Shanghai Jiao Tong University
- 3National Key Laboratory for Multimedia Information Processing, School of Computer Science, Peking University
- 4National Engineering Research Center of Visual Technology, School of Computer Science, Peking University
- 5Graduate School of Information Science and Technology, Osaka University
Overview
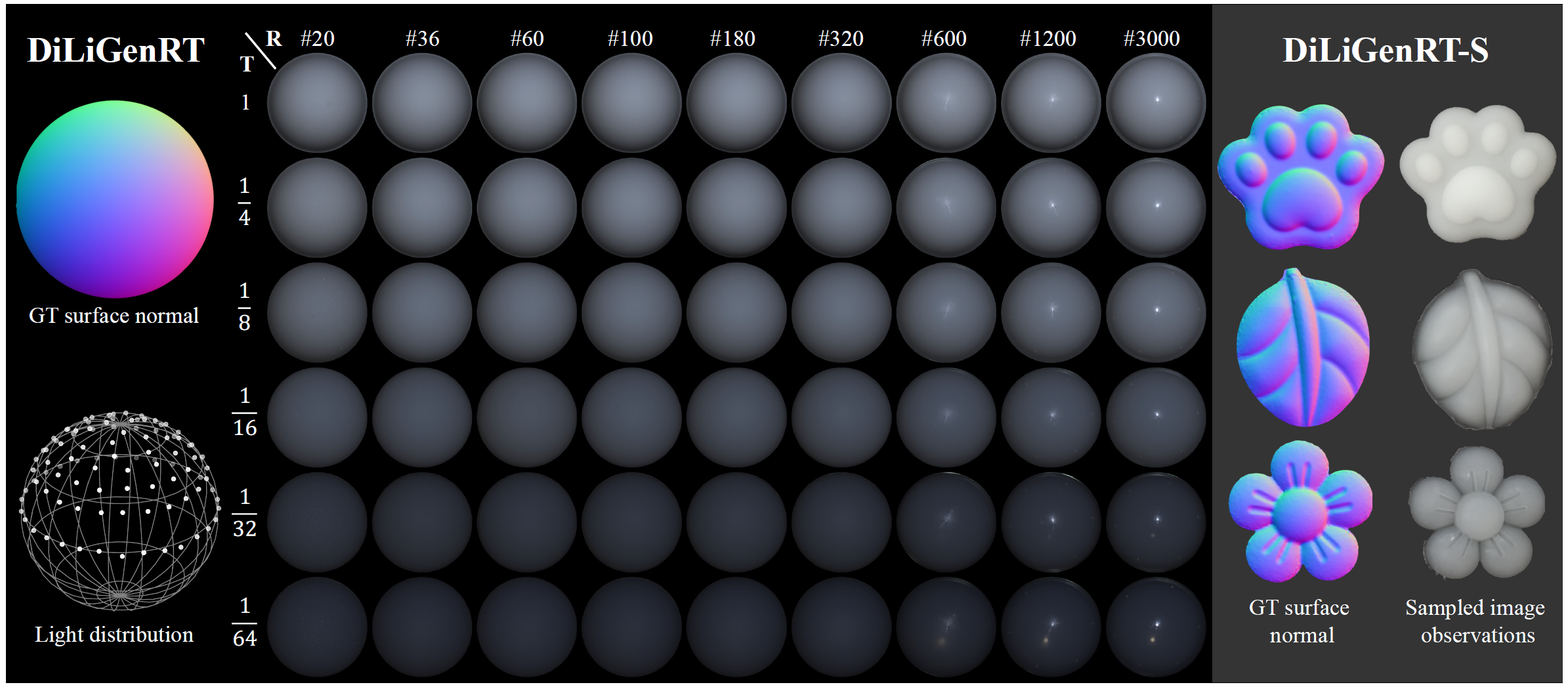
Photometric stereo faces challenges from non-Lambertian reflectance in real-world scenarios. Systematically measuring the reliability of photometric stereo methods in handling such complex reflectance necessitates a real-world dataset with quantitatively controlled reflectances. This paper introduces DiLiGenRT, the first real-world dataset for evaluating photometric stereo methods under quantified reflectances by manufacturing 54 hemispheres with varying degrees of two reflectance properties: Roughness and Translucency. Unlike qualitative and semantic labels, such as diffuse and specular, that have been used in previous datasets, our quantified dataset allows comprehensive and systematic benchmark evaluations. In addition, it facilitates selecting best-fit photometric stereo methods based on the quantitative reflectance properties.
Highlights
- First public PS dataset with quantified Roughness (9 levels) and Translucency (6 levels);
- A simple and stable process for fabricating surfaces with controlled roughness and tranlucency;
- First quantitative work space of photometric stereo w.r.t reflectance.
Febrication, Capture and `RT` Measurement
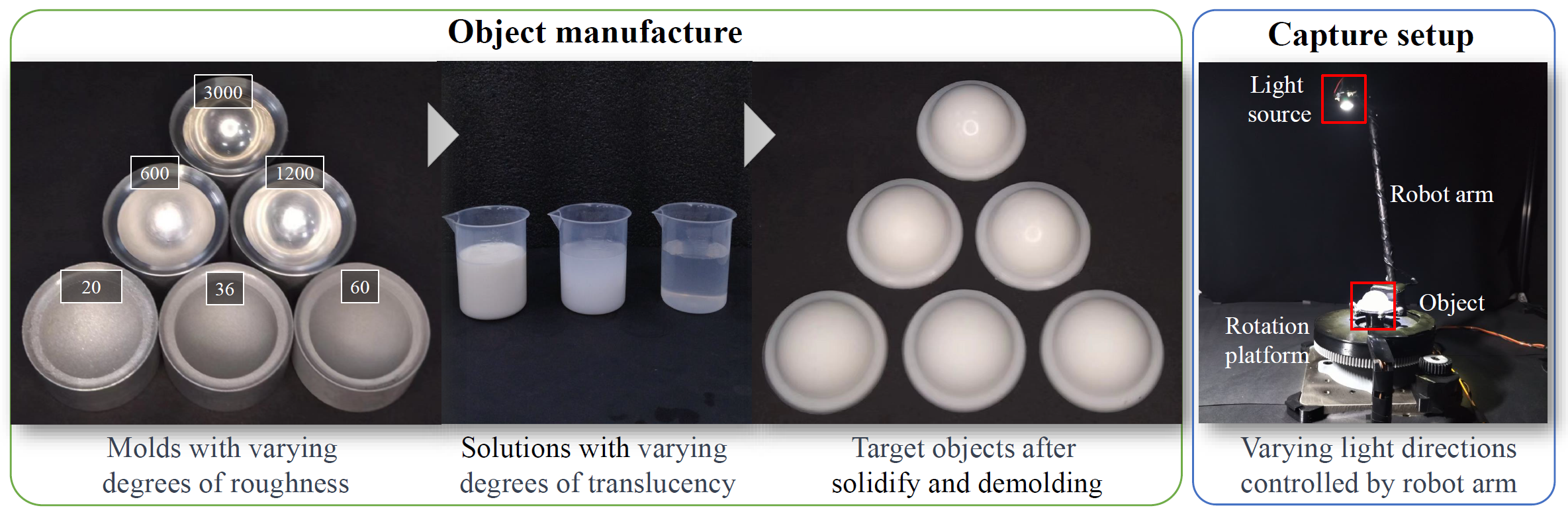
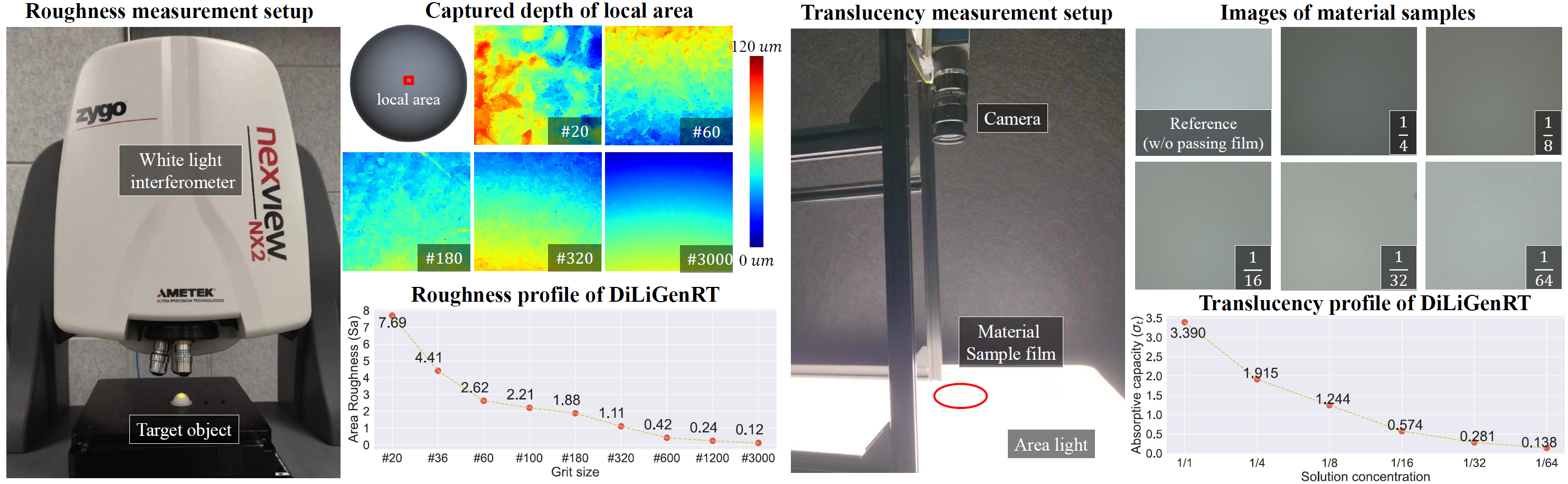
We manufacturing multiple molds with same size, and sandblasting and polish them with differen grit # (the size of granularity) to obtain diverse surface rougness. For translucecy, we mix different concentrations of pigment into silica gel to casting the molds to obtain hemi-spheres. We also take the lightweight illumination and imaging setup for capture the DiLiGenT-RT dataset. We take zygo nexViewTM NX2 to measure the accurate surface roughness of objects, and build a customerized equipment to measurement the translucency of objects.
Benchmark Results
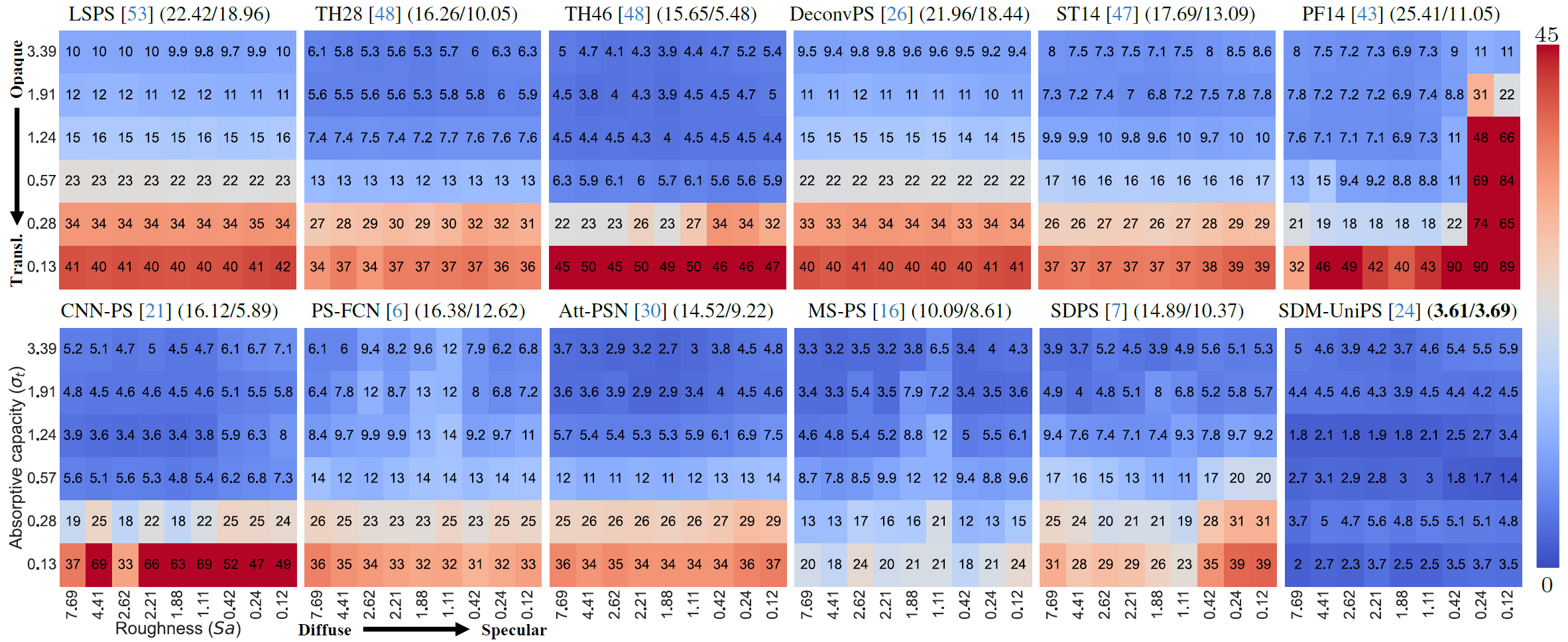
Roughness-translucency MAE matrices for non-learning-based (top) and learning-based (bottom) photometric stereo methods, showing their performance profiles under different levels of reflectance properties. The mean and median of the MAE matrix are presented near the method name. The ticks of row and column are σt (transparency) and Sa (roughness). Reducing σt corresponds to increasing translucency, while lowering Sa is associated with decreased roughness. Their error distribution matrix is visualized. More rough and less translucent samples show small reconstruction error (same as common sense).
Performance Analysis
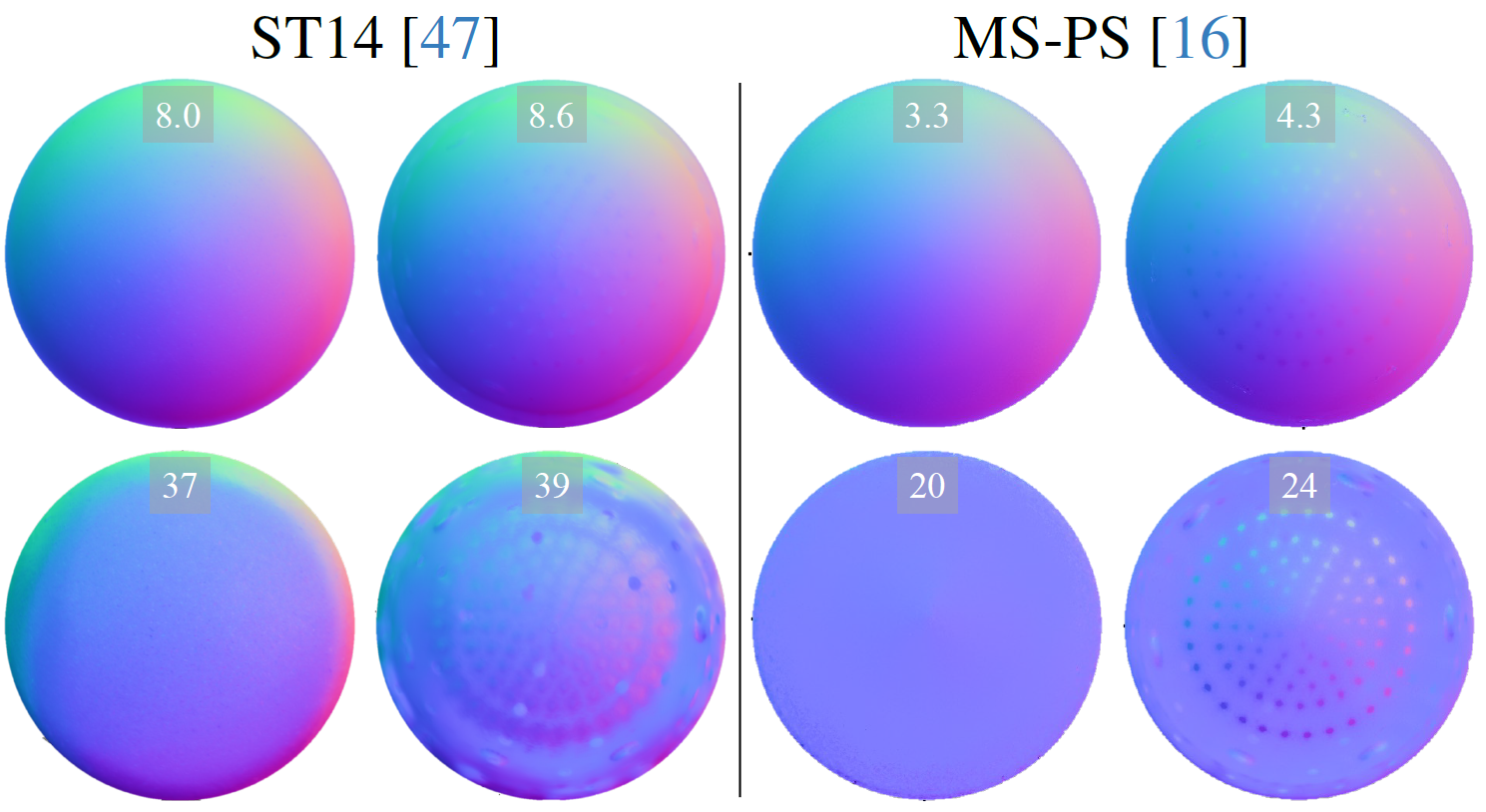
Visualization of estimated surface normals for hemisphere objects at the four corners of the translucency-roughness (top-left:most rough and least translucent, top-right: least rough and least translucent, bottom-left: most rough and most translucent, bottom-right: least rough and most translucent), which directly demonstrate the influence of roughness of translucency on surface normal estimation.
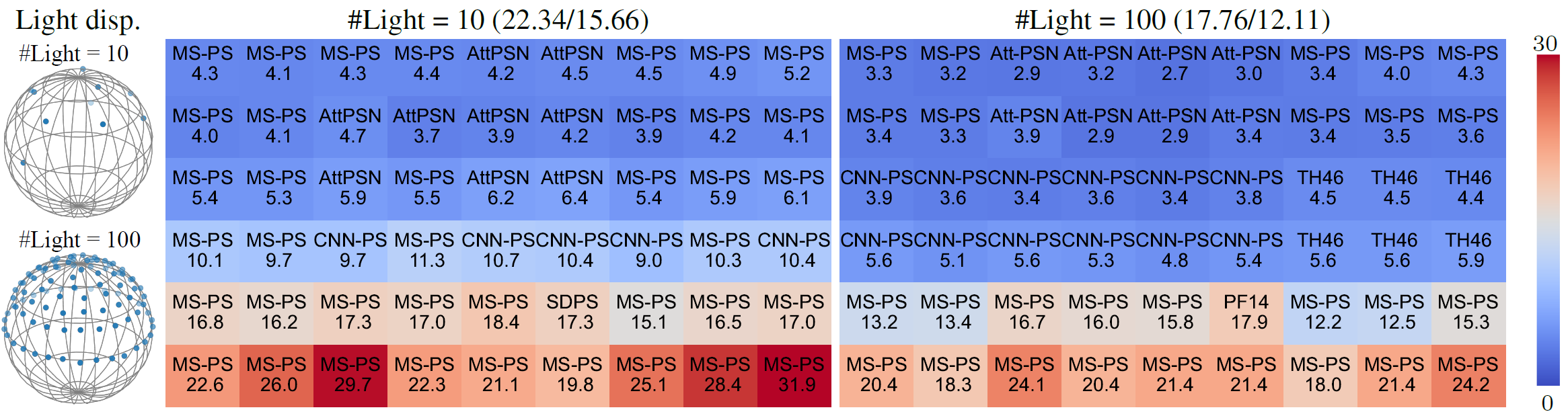
PS work space based on DiLiGenRT under sparse and dense lights (#10 and #100). Each cell records the best-performing algorithms on each roughness-translucency sample (MAE/name annotated in the heatmap block).
Citataion
@InProceedings{Guo_Ren_Wang_2024_CVPR, author = {Guo, Heng and Ren, Jieji and Wang, Feishi and Ren, Mingjun and Shi, Boxin and Yasuyuki, Matsushita}, title = {DiLiGenRT: A Photometric Stereo Dataset with Quantifed Roughness and Translucency}, booktitle = {Proceedings of the IEEE/CVF Computer Vision and Pattern Recongnition (CVPR)}, month = {June}, year = {2024}, pages = {xxxxx-xxxxx} }
Contact
Any questions and further discussion, please send e-mail to:
guoheng_AT_bupt_DOT_edu_DOT_cn.