DiLiGent-Pi: Photometric Stereo for Planar Surfaces with Rich Details –
Benchmark Dataset and Beyond
ICCV 2023 (Poster Presentation)
- Feishi Wang 1,2,3,†
- Jieji Ren 4,†
- Heng Guo 5,6,†
- Mingjun Ren 4,‡
- Boxin Shi 1,2,3,‡
- 1National Key Laboratory for Multimedia Information Processing, School of Computer Science, Peking University
- 2National Engineering Research Center of Visual Technology, School of Computer Science, Peking University
- 3AI Innovation Center, School of Computer Science, Peking University
- 4School of Mechanical Engineering, Shanghai Jiao Tong University
- 5School of Artificial Intelligence, Beijing University of Posts and Telecommunications
- 6Osaka University
Overview
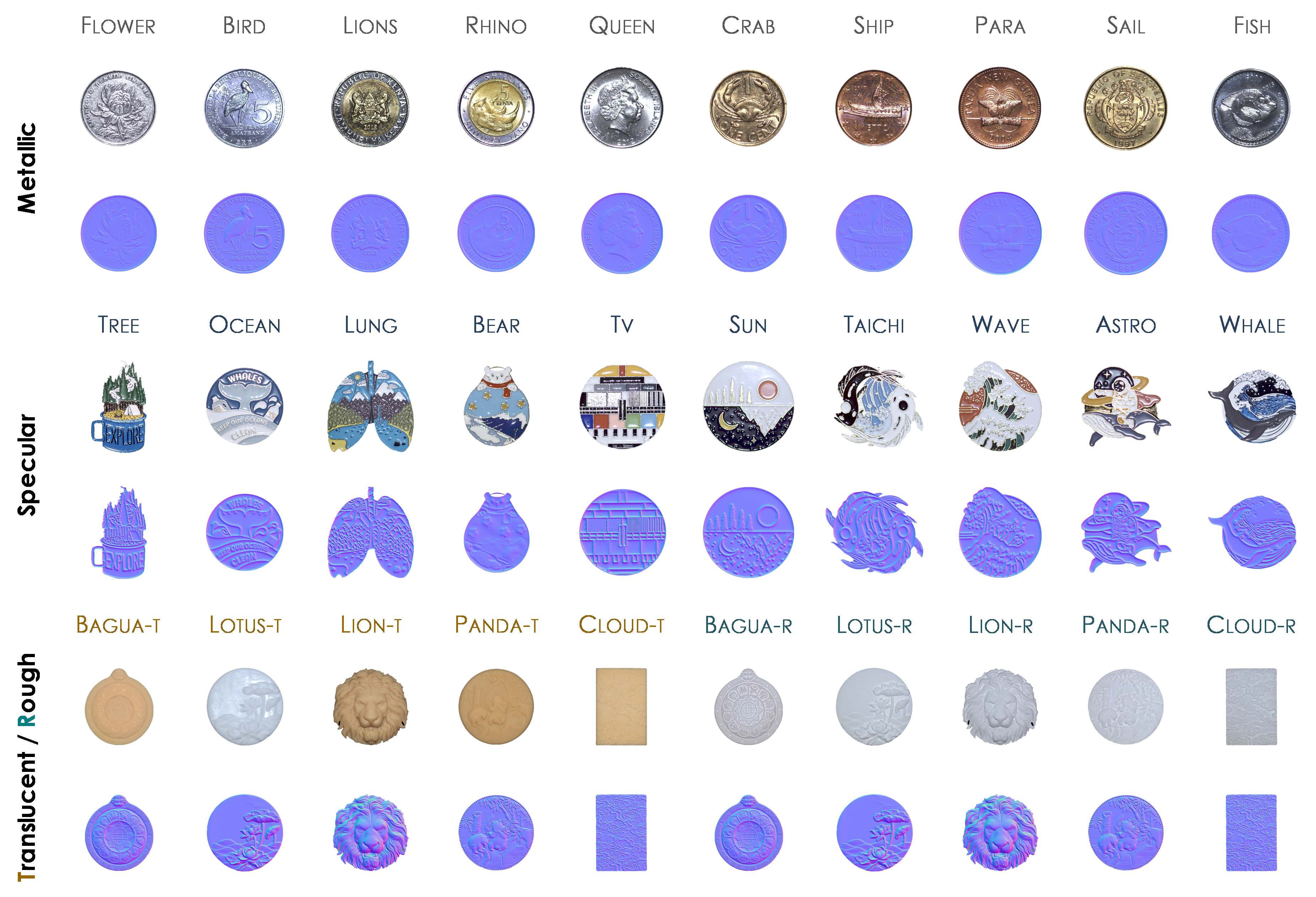
Recovering the surface details is one of the potentional advantages of Photometric Stereo over other 3D reconstruction techniques. However, there is no existing real-world datasets focus on near-planar surfaces width rich detials, which widely exist in cultural relics and manufacturing workpieces. To fill this missing part of photometrc stereo, we present a new real-world dataset DiLiGenT-Π containing 30 nearplanar objects with rich surface details. This dataset enables us to evaluate recent photometric stereo methods specifically for their ability to estimate shape details under diverse materials and to identify open problems, such as near-planar surface normal estimation from uncalibrated photometric stereo and surface detail recovery for translucent materials.
Highlights
- First public near-planar rich-details PS dataset with 30 sets of images with `ground truth` normal;
- Complex geometric surface and diverse materials are designed;
- A lightweight capture system are developed for small objects observation with omnidirectional illumination;
- Evaluating details recovery performance of PS methods and identifing new open problems.
Dateset Capture Setup and `GT` Generate Process
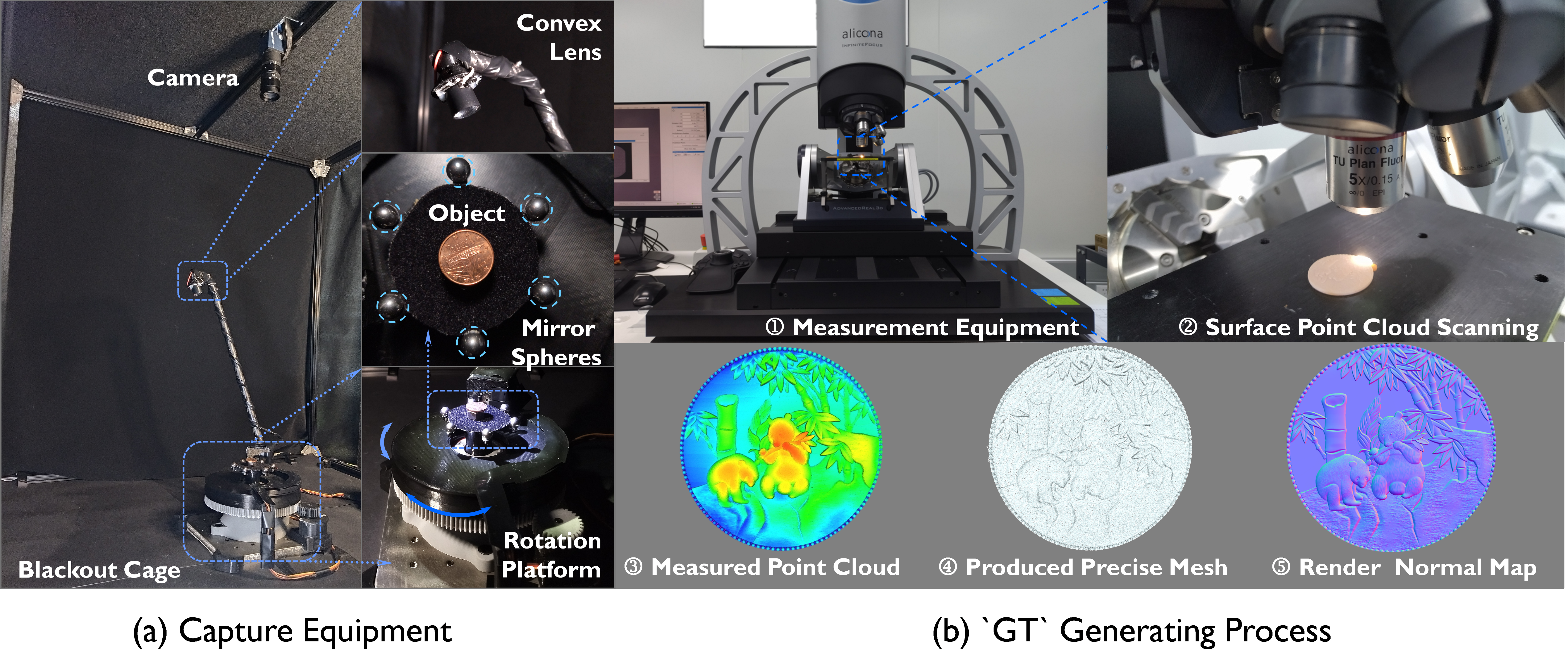
Lightweight illumination and imaging setup for capture the DiLiGenT-Pi dataset. The system is consist of two-axes rotation stage and LED for omnidirectional illumination, mirrow balls for light direction calibration, camera for image capturing, and black felt board coverd cage acts as darkroom. We take Alicona to measure the accurate surface point cloud of objects, and take the Blender to register point cloud with observed image, finally rendering the surface normal map as `ground truth`.
Benchmark Results
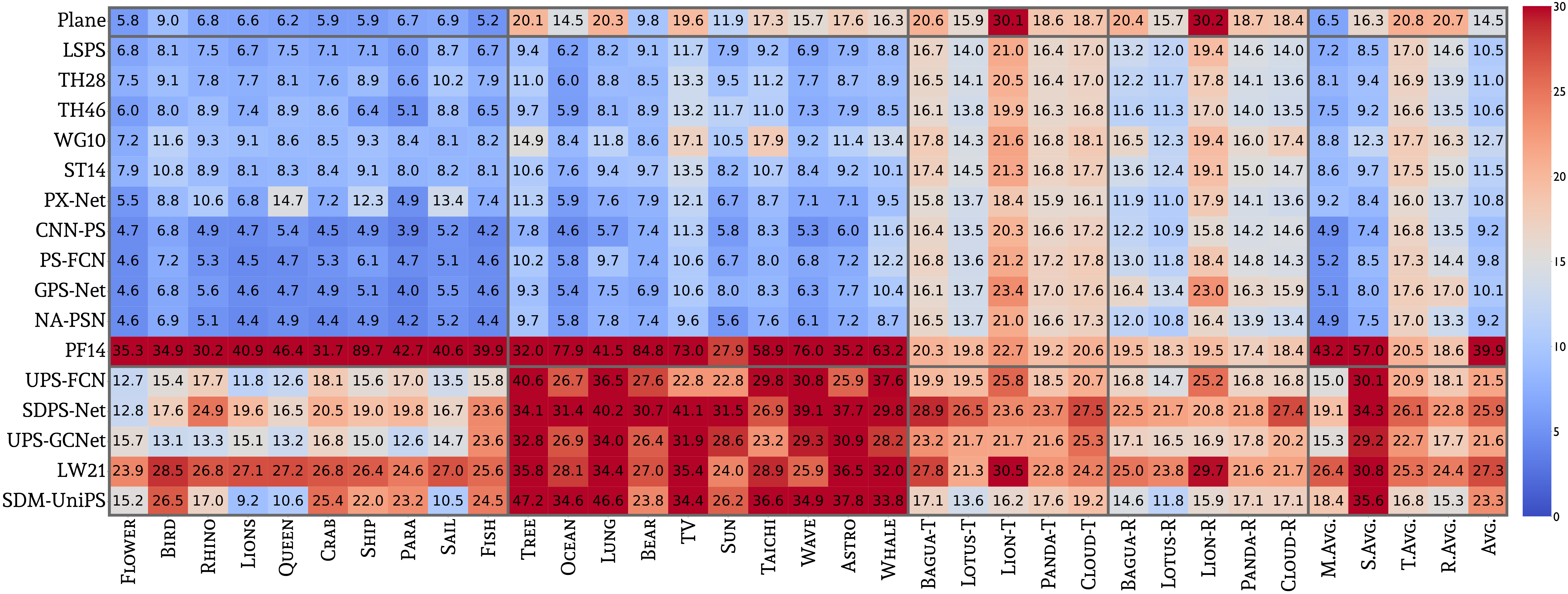
A 15*30 algo-obj error distribution matrix is visualized to depict the recovery perfoemance of each PS method.
Reconvery Performance on Selected Methods
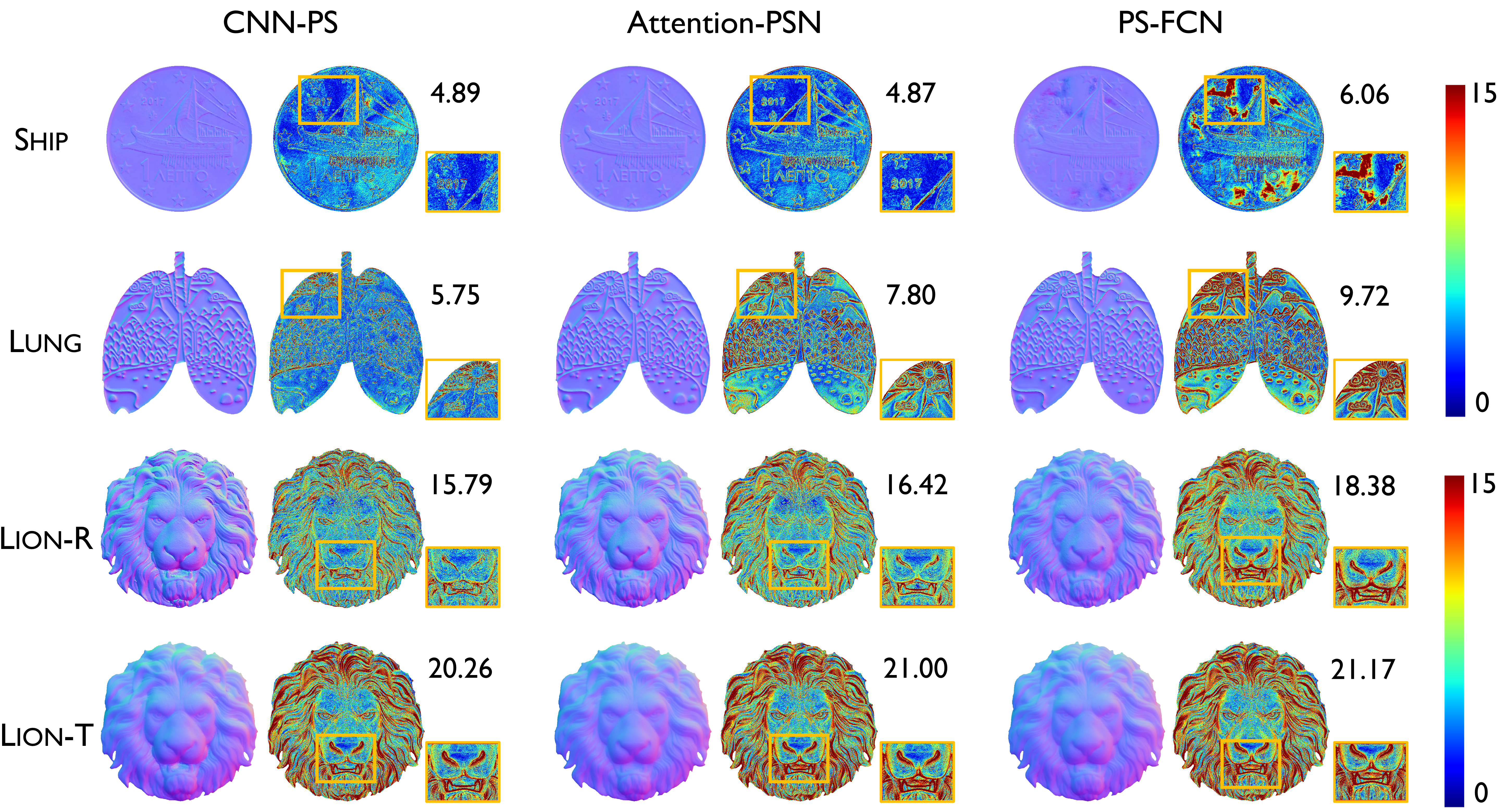
Visualization of surface detail recovery from different photometric stereo methods. Per-pixel based photometric stereo method is more effective on detail recovery compared to all-pixel based method.

Surface normal estimates of photometric stereo methods with (top row) or without (bottom row) finetuning on PS_RELIEF. Compared to calibrated method, learning-based uncalibrated photometric stereo are heavily influenced by the shape prior learned from the training dataset.
Citataion
@InProceedings{Wang_Ren_Guo_2023_ICCV, author = {Wang, Feishi and Ren, Jieji and Guo, Heng and Ren, Mingjun and Shi, Boxin}, title = {DiLiGenT-Pi: A Photometric Stereo Benchmark Dataset with Controlled Shape and Material Variation}, booktitle = {Proceedings of the IEEE/CVF International Conference on Computer Vision (ICCV)}, month = {October}, year = {2023}, pages = {9477-9487} }
Contact
Any questions and further discussion, please send e-mail to:
wangfeishi_AT_pku_DOT_edu_DOT_cn.